INDUSTRY
HR COMPLIANCE |
AI SERVICE
MACHINE LEARNING |
ML-Driven Content Recommender Predicts & Addresses HR Compliance Risks
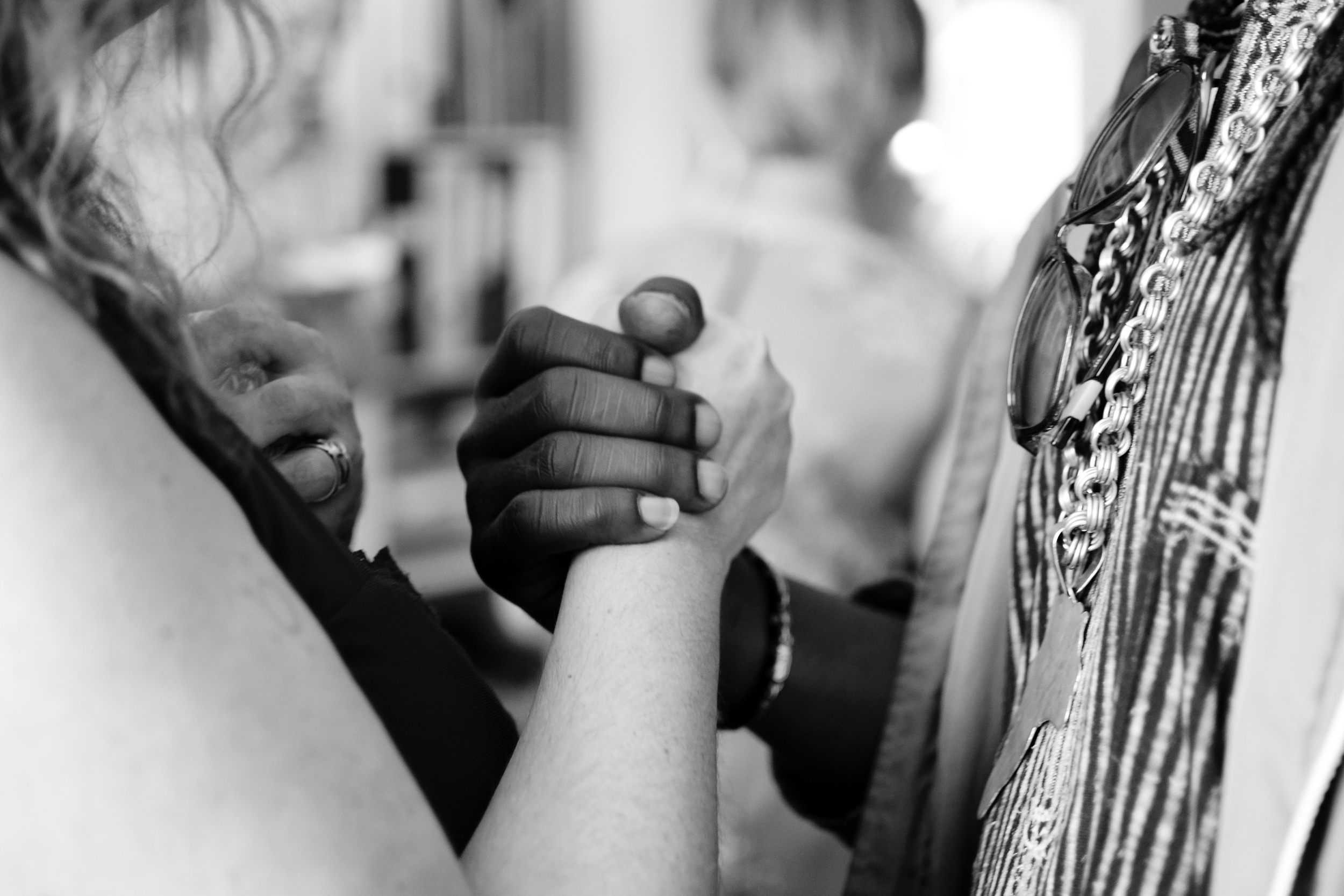
Learn how we partnered with Mineral (recently acquired by Mitratech), an HR risk management platform, to develop an ML-driven system that recommends Mineral content to Mineral customers based on their predicted HR compliance risks.
Problem:
Organizations struggle to identify their HR compliance risks because labor laws are complex, fluid and most often region-specific. Mineral wanted to predict their customers’ HR compliance risks and recommend content to point them in the right direction.
What can you learn from starting with a proof?
Proof of concept projects, also known as feasibility studies, serve a valuable purpose even when they demonstrate that a concept is not feasible. These projects mitigate risk by identifying potential challenges and preventing over-investments in non-viable ideas. Furthermore, findings from proof-of-concept projects can inform strategic decision-making. Understanding why a concept is unfeasible is the first step to making it feasible, or identifying an alternative course of action.
Let us help you improve the quality of your data →Solution:
We developed a proof-of-concept recommender that takes customer support tickets as input and returns content recommendations. The recommender uses machine learning to group companies into clusters based on similar attributes, combines support ticket content from each cluster, and extracts relevant keywords to make content recommendations.
Our hypothesis was that customers with similar attributes would file similar tickets, and the keywords in those tickets could be used to identify and recommend relevant content. However, we found that this approach needed more data than was available to be effective.
Outcome:
We determined that an ML-driven content recommender based on support ticket keywords required (i) more data and (ii) higher-quality data than Mineral could provide. We delivered actionable recommendations for Mineral to acquire and leverage such data, including data-cleaning suggestions, data-collection strategies, and advanced clustering techniques.
In the long term, the infrastructure and insights gained from this proof-of-concept project put Mineral in a favorable position to leverage customer data. By incorporating our recommendations, Mineral can enable a content recommender system that accurately predicts customer HR compliance risks and points them to relevant Mineral content.
500,000 more employers are covered before the change in law puts them at risk for being non-compliant
HUMANKIND OF IMPACT
AI IS HOW WE DO IT,