INDUSTRY HEALTHCARE |
AI SERVICE DATA-DRIVEN PRODUCT STRATEGY, AI UX & UCD, DATA SCIENCE |
Streamlining Patient Intake with Predictive Analytics Giving the Error-Prone Pen & Paper Method a Much Needed Rest
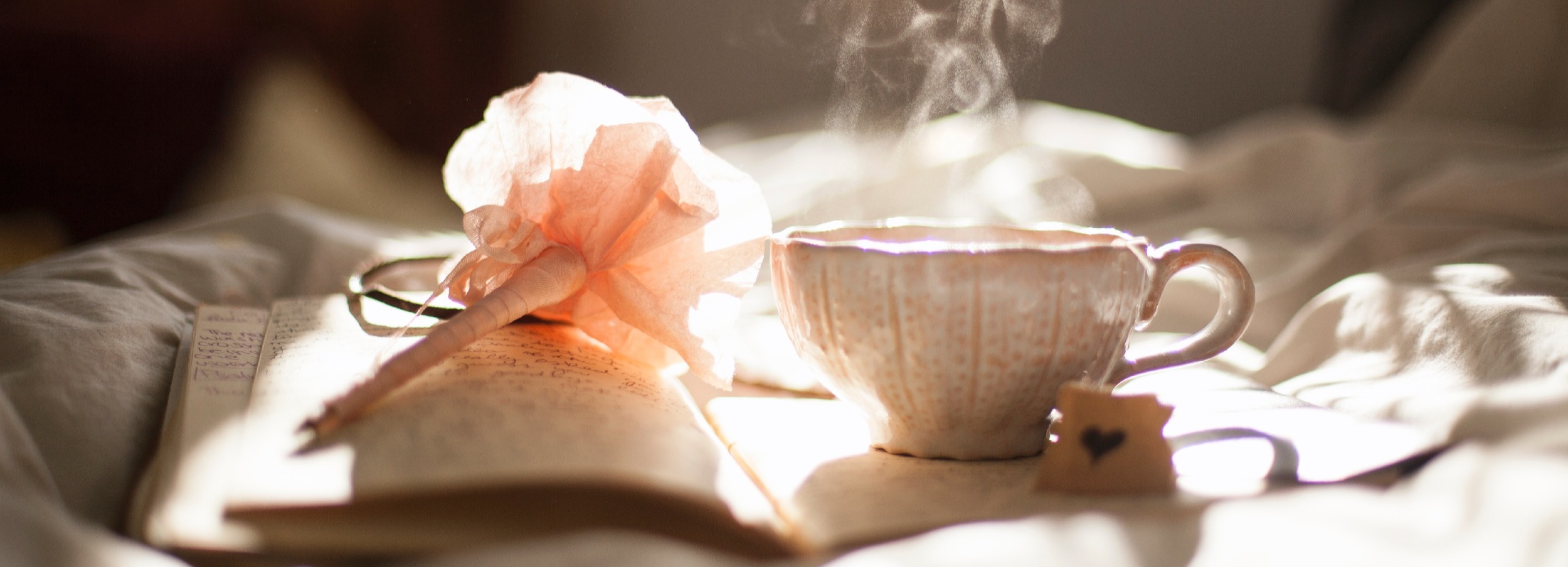
Learn how predictive analytics and natural language processing techniques were used to thwart errors, relieve intake-form exhaustion, and build a more accurate data picture for those who prefer the natural path to health and wellness.
Problem:
Today, people suffering from chronic illness not only find themselves on a quest for answers, but find themselves tethered to another lengthy questionnaire about their health. As time goes on, potentially moving from one specialist to the next, the quality of the input they provide diminishes, leading to errors and inefficiencies. This poses a significant challenge to healthcare providers who must rely on accurate information to deliver effective and do-no-harm care.
Is your business dynamic enough?
An early IBM programmer is generally given credit for the term "Garbage In, Garbage Out" to illustrate that machines process what they are given. GIGO encapsulates a fundamental truth about data handling today, but back in the 1960's when it was coined, data was seemly only handled by a few intelligent humans who not only had access to 'dumb boxes', but were detailed and accurate, able to fastidiously transform science fiction into fact: robots, satellites, a trip to the moon.
We have evolved. Quickly. Now, the smart watch on your wrist can predict if you are in A-Fib. And although we are all getting used to the unconscious understanding of GIGO and trying to provide accurate data about ourselves so that our watches do their job, what if the capturing methods are the real culprit to data quality? Predictive analytics and natural language processing techniques can be leveraged to optimize data intake forms and improve data quality. By dynamically selecting questions based on previous responses, you can reduce the time humans spend filling out forms and ensure accurate and complete data is collected. A gold rush for chronic patients navigating our healthcare systems, but also prudent for any organization who need to collect higher quality data where big decisions are at stake. So if you're headed to the moon, consider if your business, and your data, is dynamic enough for a successful voyage.
Solution:
Our team developed a statistical model that computed associations among patient responses. The model was then used to drive question selection dynamically based on intake responses given so far. We went on to craft a proof-of-concept (POC) user experience for demonstration, which allowed us to test the efficacy of our solution and gather feedback from practitioners in the field.
Our statistical model used predictive analytics to omit irrelevant intake-survey questions on a patient-to-patient basis. This adaptive approach improves patient experience by reducing time spent filling out intake surveys. Additionally, it ensures that frustration and fatigue do not undermine response/data quality.
Outcome:
The AI solution streamlined patient intake forms by using statistical associations among patient responses to guide question selection. Patients reported feeling that the model-driven intake survey “understood them”; they put more effort into their responses, so our client collected higher quality data. Our approach to combine a data-driven algorithm with a POC that facilitated efficacy testing the UX, allowed for the integration of our model into the client’s electronic medical record (EMR) technology for provider use. If reducing frustration and fatique makes us feel like we could fly to moon, it might be safe to PREDICT that our responses to dynamically produced surveys also improves the quality of our answers - and the quality of our care.
HUMANKIND OF IMPACT
5,000,000 chronic patients across 10,000 practices may finally feel understood.
9,000 more people each year will be able to start their new careers in America.
HUMANKIND OF IMPACT
AI IS HOW WE DO IT,
humanity
is why we do it