INDUSTRY HEALTHCARE |
AI SERVICE ML MODELING & MLOPS |
Protecting Privacy in Clinical Trial Data with AI-driven Anonymization Allows Researchers to See the Future
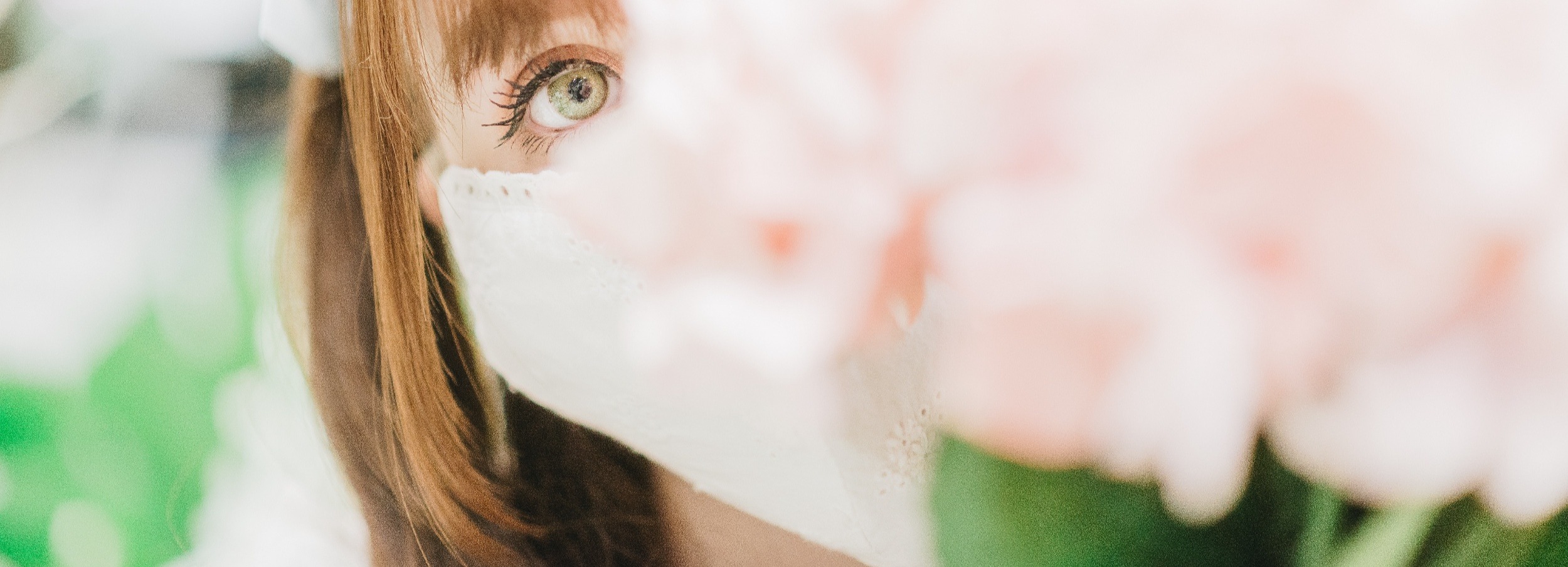
Learn how using machine vision to ensure data privacy for clinical trial participants was also preserving data integrity for researchers.
Problem:
An international pharmaceutical firm performing innovative medical research needed an AI solution to anonymize Personally Identifiable Information (PII) from clinical trial data, specifically medical images, to ensure privacy for their participants, while maintaining data integrity for their research.
How can AI find you and make you anonymous?
Aggregation, masking, generalization, perturbation, there are several techniques that can be used to anonymize data. Finding and anonymizing data within a dataset involves several steps to ensure that data is de-identified and cannot be linked back to specific individuals. Achieving true anonymity in a dataset is challenging and requires careful consideration of legal, ethical, and technical factors. But once data has been effectively de-identified and cannot be linked back to specific individuals, you must undergo statistical methods and other techniques to assess the risk of re-identification. In the case of clinical studies, people must be protected, research practices must be ethical, laws must be followed, bias eliminated, and data must be able to be shared. Anonymity facilitates data sharing and promotes collaboration among researchers. When data is anonymized, it can be shared more easily with other researchers, which can lead to increased scientific transparency, reproducibility, and advancement of knowledge. There are many good reasons to be both findable and remain anonymous.
Solution:
We developed a set of machine vision models to anonymize various aspects of clinical trial data. These models were trained using publicly available datasets, ensuring a robust framework for addressing PII concerns in clinical data.
This solution selectively anonymizes medical images, removing PPI while also preserving the images’ research value. It allowed our client to ensure data privacy for clinical trial participants without jeopardizing the validity of their research.
Outcome:
The client received a set of AI models with promising performance metrics, demonstrating their effectiveness in anonymizing clinical trial data, ensuring privacy, and maintaining the integrity of the research.
HUMANKIND OF IMPACT
64,800 patients remain anonymous to offer others a chance to get better.
9,000 more people each year will be able to start their new careers in America.
HUMANKIND OF IMPACT
AI IS HOW WE DO IT,
humanity
is why we do it